Recommended Blogs
Generative AI for Observability: Revolutionizing System Performance Monitoring
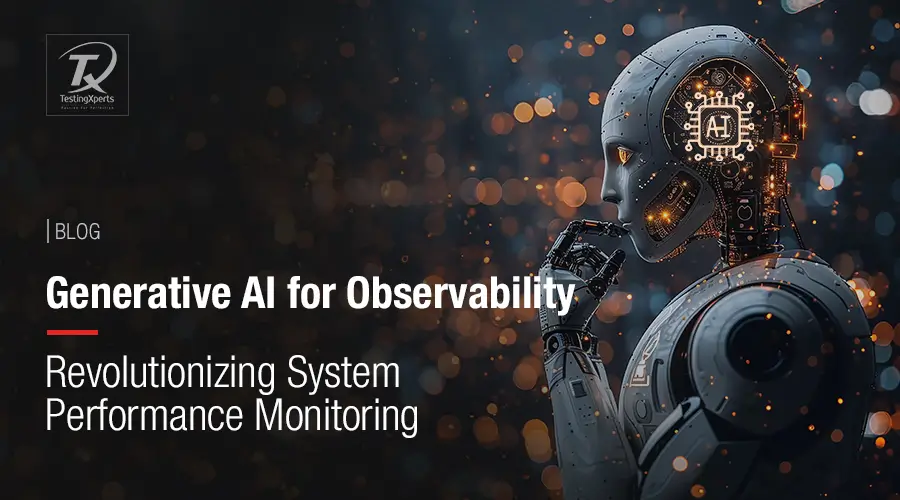
- The Shift to Generative AI for Observability
- Why Generative AI in Automation Observability?
- Key Advantages of Generative AI for Observability
- Generative AI in Action
- Conclusion
- Why Choose Tx for AI in Observability
“By 2025, 75% of organizations will shift from piloting AI to operationalizing it at scale.” – Gartner
The digital world is rapidly evolving and so are the expectations from IT infrastructure. As enterprises strive to maintain seamless operations, the need for real-time system performance monitoring has reached an all-time high. In this ever-complex landscape, traditional observability tools are proving insufficient to keep up with the scale, velocity, and intricacy of modern applications. Enter generative AI – an innovation that’s revolutionizing the foundation of observability.
The Shift to Generative AI for Observability
For years, observability has been paying attention on gathering data through logs, trace and metrics, with engineers manually observing this information to recognize issues, optimize performance, and ensure system health. Traditional observability tools can monitor system performance, but they often need significant human intervention to analyze the data, make decisions, and act upon them. This approach can be slow, flawed, and inefficient, specifically in today’s multi-cloud, containerized, and microservices-based environments.
At its core, generative AI is about enabling machines to understand patterns, generate new content, and make predictive decisions autonomously. In terms of observability, it changes the reactive, manual nature of the system monitoring into a proactive, automated process that predicts bottlenecks and offers AI testing solutions.
Why Generative AI in Automation Observability?
Think of an IT team, in charge of monitoring a huge infrastructure supporting millions of users worldwide. Traditionally, system health would be checked using dashboards filled with data – CPU usage, disk I/O, memory consumption, and network latency. When some anomaly occurs, like an unexpected spike in CPU usage, alerts flood the system, needing engineers to sift through endless logs to diagnose the key cause. This often causes alert fatigue, where important issues can be overlooked considering the large number of notifications.
Now, think of a generative AI system enclosed within this environment. The AI actively monitors the systems from historical data to understand what it pictures as normal or abnormal behavior. When an anomaly is detected, it just alerts the team but predicts the potential impact it may have. This may suggest remedial actions before the situation escalates. This shift from reactive to proactive monitoring reduces downtime significantly and perks up the overall system performance.
Key Advantages of Generative AI for Observability
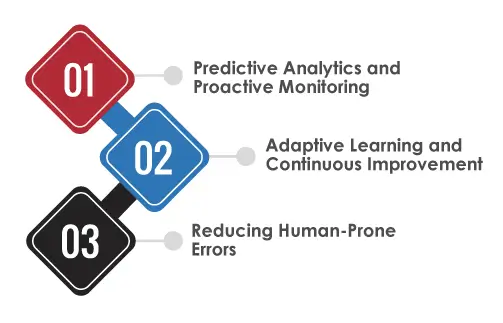
1. Predictive Analytics and Proactive Monitoring
Generative AI’s most important contribution to observability is its capacity to predict issues before they occur. Traditional observability tools are often reactive – they alert the engineers once an issue has occurred. In comparison, generative AI analyzes the historical data to recognize patterns that precede failures, enabling predictive monitoring.
For instance, in a cloud-based application running thousands of microservices, generative AI can foresee when a specific service will run out of resources based on the past usage patterns. It can then suggest scaling up resources or reconfiguring the infrastructure to avoid performance degradation.
2. Adaptive Learning and Continuous Improvement
Generative AI systems learn and improve over time. Unlike static monitoring tools, generative AI adapts to changes in system behavior and infrastructure. For example, as a business scales its operations and deploys new microservices and updates its cloud architecture, generative AI regularly learns from new data to optimize its predictions and recommendations.
This adaptability is critical in dynamic environments where changes occur rapidly and frequently. By regularly learning, generative AI ensures that monitoring remains effective and relevant, even when the system evolves.
3. Reducing Human-Prone Errors
In traditional observability models, a lot of the monitoring and incident resolution relies on human experience. However, this human intervention often leads to errors – be it due to misinterpreted data, delayed response times, or the cognitive load of managing huge infrastructures.
Generative AI, with its ability to automate most of the decision-making process, eradicates these risks. By autonomously analyzing system performance and offering precise recommendations, AI-driven observability reduces the chance of human-error, leading to much more reliable system performance.
Generative AI in Action

eCommerce Application Performance
Think of a global eCommerce platform that handles millions of transactions daily. Earlier, monitoring this system needed engineers to check the logs for transaction errors, unexpected traffic spikes, and server slowdowns during peak sales events.
With generative AI, the system can automatically predict when server resources will be strained due to an influx of traffic and suggests scaling up infrastructure in advance. In addition to this, if an anomaly occurs, like a sharp increase in checkout errors, the AI can pinpoint if the issue lies with the payment gateway, the database, or the user interface, reducing resolution time drastically.
Financial Trading Systems
Financial trading platforms shall operate with near-zero downtime, and even a small delay can lead to significant financial losses. Traditional monitoring systems are reactive, which means they by the time an issue is identified, already have caused substantial damage.
Generative AI helps by regularly learning from trade volumes, market fluctuations, and transaction latencies to predict potential system slowdowns or failures. In doing so, it allows the platform to adjust resources in real time, making sure consistent performance even during high-volume trading periods.
Conclusion
Generative AI in Observability is not just a buzzword – it’s a transformative technology poised to revolutionize how organizations monitor, manage and optimize system performance. Enabling proactive monitoring, predictive analytics, automated root cause analysis, and continuous learning, generative AI significantly enhances the observability landscape, driving business continuity and operational efficiency.
Why Choose Tx for AI in Observability
Tx is leveraging AI to redefine observability, offering cutting-edge solutions empowering businesses to optimize system performance and reduce downtime. Our AI-driven observability tools go beyond traditional monitoring by offering predictive analytics, automated root cause analysis, and real-time insights, delivering proactive management of complex infrastructures. With a thorough understanding of the modern challenges like scalability, multi-cloud environments, and microservices, Tx’s solutions are customized to meet the specific needs of your organization. This helps you stay ahead of the issues before they leave any impact on your operations.
Trusted by industry leaders, Tx combines innovative technology with expert consulting to deliver unparalleled system reliability and performance.
Discover more
Stay Updated
Subscribe for more info