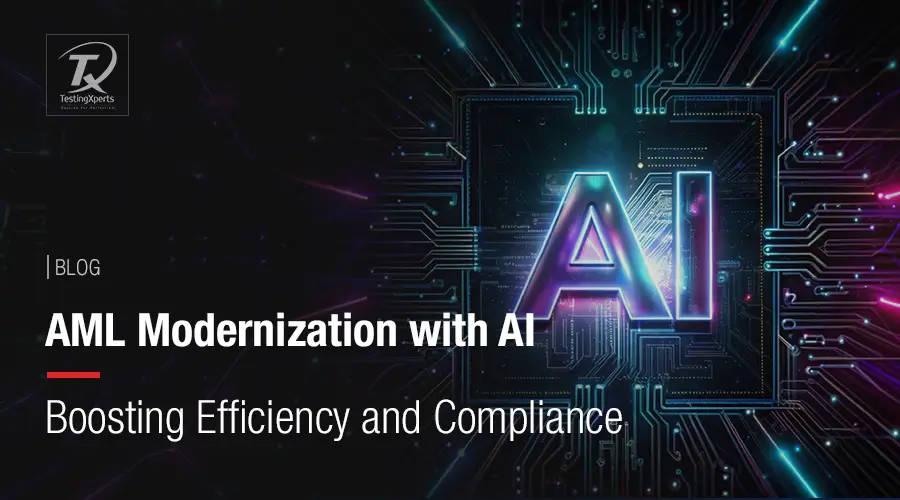
- What is AML Modernization with AI?
- Drawbacks in Traditional AML Transaction Monitoring
- Benefits of AML Modernization with AI
- 5 Use Cases of AI in Anti-Money Laundering
- How can Tx help with AML Modernization with AI?
- Summary
Financial crime management has been a significant challenge over the last few decades. Although regulatory bodies worldwide have introduced several measures and guidelines to tackle money laundering activities, there are still considerable challenges to ensure compliance with the finance crime guidelines. Also, the traditional rule-based system is not enough to detect money laundering activities because of the involvement of new technologies like AI, robotics, etc. There’s a long history of financial institutes facing difficulties in establishing and maintaining a Financial Crimes Compliance (FCC) program and ensuring that it maximizes productivity and is client-friendly and cost-efficient.
Some Anti-Money Laundering (AML) fines in 2024:
- Deutsche Bank was fined $186 million for inadequate AML controls despite receiving warnings from regulators.
- Commerzbank was fined €1.45 million for failing to update customer data and put in place enough security measures.
- HMRC revealed that estate agency businesses were fined over £1.6 million for failing to register or re-register for AML compliance.
This doesn’t come as a surprise, as criminals are becoming more professional in evading controls, and banks find it challenging to pinpoint real money laundering actions. This is why financial institutes have started to replace traditional AML transaction monitoring software with smarter AI-enabled software. This level of AML modernization with AI would assist in finding hidden patterns in payments and connections between sender and receiver. The result could be fewer false positive alerts, improved security protocols and regulatory checks, and reduced compliance costs. But how do we make all this possible?
What is AML Modernization with AI?
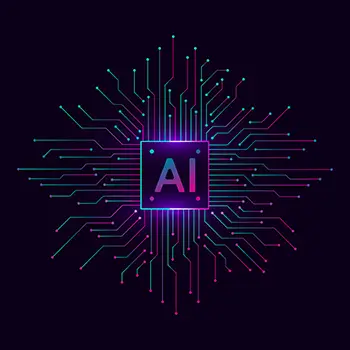
Certain factors make up a go-to anti-money-laundering (AML) process, which involves:
- An enterprise-wide comprehensive risk assessment
- Meeting the traditional AML compliance pillars (internal controls, training, sufficient resources, and testing)
- Developing a detailed governance framework consisting of comprehensive policies and procedures, security metrics, and risk committee team members details
- Strictly implementing regulatory standards and compliance.
The AML base depends on three critical, resource-intensive, and complex processes – Sanctions Screening, KYC, and Transaction Monitoring (TM). The concerned authorities working on these processes have to deal with the tedious task of gathering, inserting, and organizing data from various external and internal sources, assessing risk, analyzing data, discarding hundreds of false positives, and creating detailed reports. However, when a disturbance in the process or an uncalculated issue arises, assigning more human resources is not the right choice. Instead, integrated AI-based tools and technologies to ensure the proper working of the FCC program per industry standards and best practices would be the best option.
AML modernization with AI involves automating some aspects of TM, KYC, or alert analysis screening to speed up productivity and remain available 24/7 without getting tired. It offers a level of automation and reduces dependency on manual processes, allowing businesses to restructure their delivery operational models, including offshore, nearshore, and outsourced models traditionally leveraged by them. Also, GenAI, a subset of AI systems trained using large datasets to create new content and consists of technologies like LLMs, can deliver value for FCC systems, especially when businesses have to analyze, pare, and summarize unstructured data.
Drawbacks in Traditional AML Transaction Monitoring

The traditional AML process involves running routine scans for transactions that depend on pre-defined flags and rules for future investigation. When these rules generate alerts, the bank operations team investigates whether they are false positives or suspicious transactions. Even though this process has been running for so many decades, there were certain drawbacks that make it unsuited for the modern business ecosystem. Let’s look at some of the drawbacks of traditional AML transaction monitoring:
New Scenarios Remain Undetected:
Since the arrival of innovative solutions like AI, ML, cloud, RPA, etc., the tech landscape has shifted, and fraudsters have found new ways and techniques for money laundering scams. This is why companies have to constantly evaluate transactions, external factors, and new scenarios, which is not possible with traditional transaction monitoring. Even the slightest delay can result in non-compliance issues and substantial financial losses.
High Operational Cost:
According to statistics, 90-95% of the time, traditional AML solutions generate false positive alerts. These alerts require careful analysis to prevent non-compliance in identifying and reporting fraudulent transactions. Also, the process will need an adequate workforce depending on the volume of alerts generated and the bank’s size. They have to waste time and resources analyzing these alerts and taking appropriate actions.
Identifying New Fraud Scenarios:
This process involves leveraging human analysis of past events to identify new patterns in transactional fraud. It requires tedious manual work, and some scenarios remain unnoticed due to a lack of robust data analysis.
Even if banks take all these measures, the possibility of undetected suspicious transactions in traditional AML will always be high. Banks must shift towards AML modernization by leveraging AI and ML for transaction monitoring to overcome this dilemma.
Benefits of AML Modernization with AI
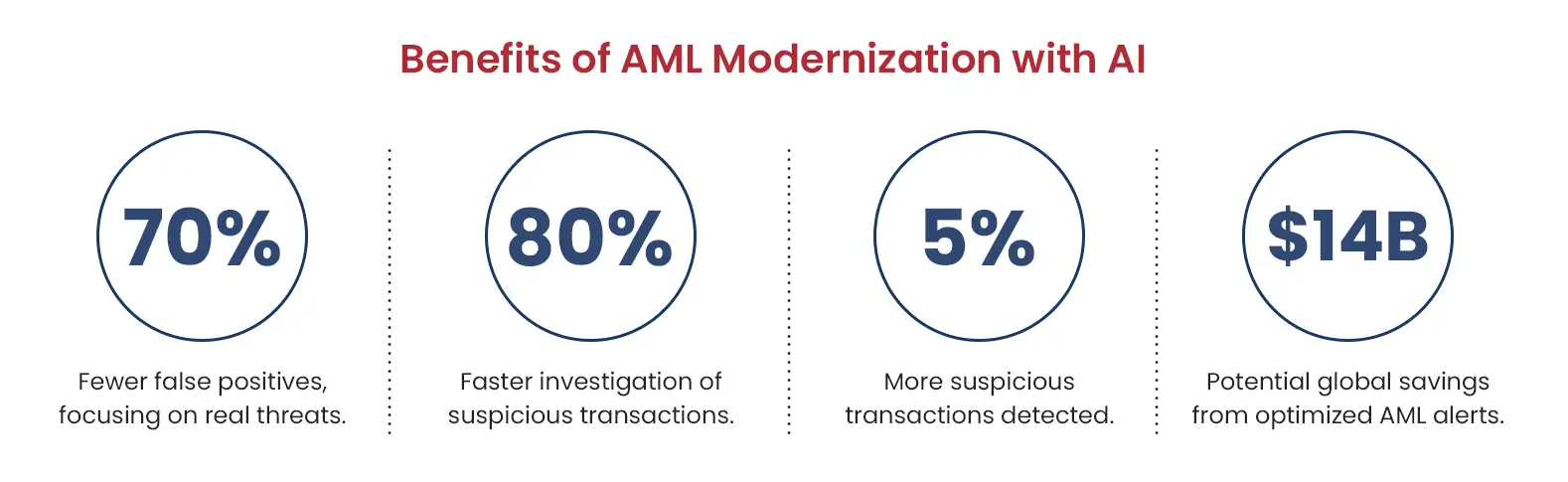
Banks must adjust their security measures for the activity to raise red alerts. If very few alerts are generated, they can miss the chance to catch criminal activity, which would attract regulators’ attention and hefty fines. On the other hand, too many alerts could sweep away compliance staff, as they must analyze each alert and decide how to proceed. With AI integration, banks can generate approximately the same number of SARs (suspicious activity reports) but with low false positive rates. Let’s take a look at some of the benefits of AML modernization with AI:
Low Operational Cost:
AI-enabled anti-money laundering solutions can reduce operational costs by controlling false positive alerts, which compliance teams must analyze and investigate. As each alert triggers a level-1 investigation, it is better to adopt an AI-based approach to only escalate level-2 or higher investigation to concerned authorities.
Optimize Risk Detection Capabilities:
AI solutions help optimize the risk detection capability of AML systems by analyzing large volumes of structured and unstructured data. They assist risk assessment teams in identifying transaction patterns to detect anomalies. For instance, neural patterns can identify patterns similar to the ones they have been trained in and raise alerts when criminal activity (based on the same rules) is detected.
Better Compliance and Governance:
IT organizations, businesses, and the banking industry are slowly changing AML regulations and global rules. Regulators in the US and UK have asked banks to integrate AI and ML solutions within their AML mechanisms and optimize risk-identification tests. This would lead to better detection of money laundering activity, reducing regulatory scrutiny.
5 Use Cases of AI in Anti-Money Laundering
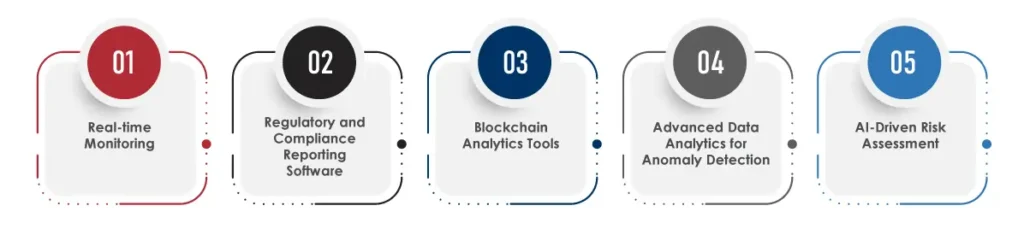
Leveraging AI in AML systems would optimize risk management by offering enhanced detection, remediation, and reporting mechanisms for money laundering operations. This would also ensure adherence to strict regulatory practices and regional and international laws and integrity within the transaction process. To better understand the role of AI in anti-money laundering, let’s look at some of its use cases:
Real-time Monitoring:
One of the significant aspects of AI in AML is that it facilitates real-time monitoring, detection, and analysis. This helps prevent possible scam activity by detecting it and initiating an early investigation. For instance, Standard Chartered Bank leverages AI-based solutions in risk-free transaction procedures. It helps them monitor all transactions in real time, and if fraudulent activity is detected, it will send an instant alert.
Regulatory and Compliance Reporting Software:
AI-powered compliance solutions would allow financial institutes to manage the complexities of regulatory and compliance reporting effectively. AI technologies would ensure that the AML systems comply with all legal rules by supporting due diligence and reporting any non-compliance fines.
Blockchain Analytics Tools:
These tools are becoming a crucial asset in AML surveillance for digital assets like cryptocurrencies, online payments, etc. Financial institutes can easily monitor and flag suspicious transactions by leveraging blockchain analytics tools. These tools also offer a 360-degree view of how cryptocurrency transactions are flowing.
Advanced Data Analytics for Anomaly Detection
AI/ML developments and Big Data Analytics in the AML compliance ecosystem make it possible to pinpoint complex patterns and abnormalities that might cause money laundering incidents. By leveraging data from multiple sources, these technologies allow financial institutes to make better decisions and prevent illegal activities.
AI-Driven Risk Assessment:
AML modernization with AI has contributed to optimizing risk assessment by integrating analysis with a broader scope. AI-driven AML compliance solutions offer a detailed insight into money laundering risks and provide appropriate solutions to monitor these activities digitally. Banks can process large datasets quickly to analyze patterns indicating the chances of money laundering or other finance crimes. For instance, CitiBank leverages AI to support its risk management process as it deals with transactional and behavioral patterns globally. The pattern recognition feature allows them to assign scores to the identified risks such that the compliance teams can address alerts with high priority.
How can Tx help with AML Modernization with AI?
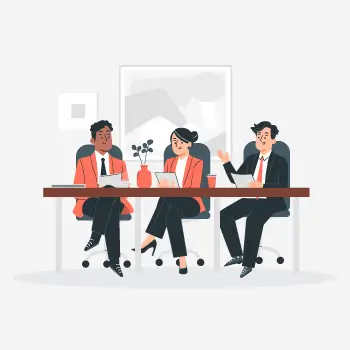
AML modernization with AI requires a strategic approach and an ecosystem of technology partners, including AI and GenAI solutions experts. The process involves planning, designing, building, testing, and maintaining the systems with minimal risks. The AML modernization will emphasize AI adoption, allowing financial institutes to cut costs, maximize transparency, and ensure compliance with AML laws. But to ensure the successful integration of AI solutions, one must partner with a reliable QA partner for robust AI testing.
Partnering with Tx gives you access to our next-gen QA services, which combine AI and RPA-based low-code/no-code automation to deliver high-quality financial solutions. For your AI-related AML modernization project, we will leverage our in-house framework for model evaluation and validation, API testing, UX testing, compliance audit, data quality management, and interoperability to ensure the ethical use of AI systems. We have established banking TCoE to manage the entire testing working with project stakeholders. Our in-house accelerators Tx-SmarTest, Tx-ReUseKit, Tx-PEARS, Tx-UiPath, and Tx-Automate would ensure robust and quicker testing outcomes while ensuring 30% QA cost savings.
Summary
AML modernization with AI transforms how financial institutions tackle money laundering, enhancing efficiency and compliance. Traditional AML systems often struggle with high operational costs, false positives, and undetected risks, making them inadequate for today’s complex financial crimes. AI-driven solutions address these challenges by automating processes like transaction monitoring, KYC, and alert analysis, leading to improved detection of hidden patterns and reduced false positives. AI’s ability to analyze vast data sets enables better compliance and more precise fraud detection, ensuring adherence to global regulations.
Partnering with Tx provides financial institutions cutting-edge AI and RPA-based QA services to ensure effective and seamless AML modernization, maximizing transparency and minimizing risks. To know how Tx can assist you, contact our experts now.